New Profs: Anthony Stein
He brings artificial intelligence to the field [13.01.23]
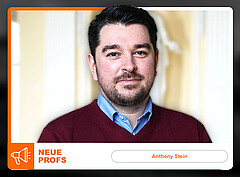
[Translate to English:] Jun.-Prof. Dr. Anthony Stein | Foto: Corinna Schmid (verändert)
Whether it's weed control by robots or crop yield prediction - digitalisation is also advancing in agriculture. Jun. Prof. Dr Anthony Stein is at home in this world: he has been head of the Artificial Intelligence in Agricultural Engineering department at the University of Hohenheim since 2020.
Artificial intelligence, often abbreviated to AI, is far more than just machine learning. Rather, this discipline is very multifaceted, the computer scientist enthuses. He encourages students to dare to tackle such supposedly complex topics - they can certainly be demystified.
Mr Stein, you have been at Hohenheim since March 2020, so you are actually a long-established Hohenheimer. But because of the pandemic, no interview with you has yet appeared in our "New Profs" series. How was your start back then, practically at the same time as the beginning of the crisis?
Hinweis der Redaktion |
Seit Beginn der Corona-Pandemie war es zeitlich nicht mehr möglich, die traditionellen Willkommensinterviews mit neuen Profs durchzuführen. Nun wird dies in Form einer Serie mit schriftlichen Fragebögen nachgeholt. |
Surprisingly well. Although all areas had to adjust, I never had the impression that I received necessary help or important information late or not at all. I was very positively surprised. Moreover, I was very warmly welcomed by my colleagues at the Institute and immediately had the feeling that I fully belonged.
The only thing I had to do without was the first handshake, which I had appreciated so much until then. I had also always imagined that I would drink a lot more coffee outside my own institute when starting out as a professor at a new university, e.g. when getting to know colleagues :-)
Your professorship is one of 10 junior professorships on AI funded by the state in 2020. What exactly does AI in agricultural engineering involve?
Lots. Agricultural engineering, like pretty much every field, is subject to ongoing digitization. Accordingly, in almost all process engineering and other agricultural processes, data is becoming measurable and information is being captured that can be analyzed and processed by intelligent software - colloquially known as AI.
A concrete example is weed control by means of autonomous robots that can detect weeds on the basis of AI and control them with the utmost precision - and only if this is at all necessary from an agronomic point of view. But you can also look for other examples on higher scales, away from the field, so to speak, such as predicting crop yields based on satellite imagery in combination with weather and crop development data as well as historical yields.
What fascinates you about the subject?
The multifaceted nature of this discipline. Contrary to the often perceived misconception, AI is in fact much more than "just" machine learning with deep artificial neural networks (deep learning). Since the 1950s, AI has been concerned with the solution of complex search problems, the explicit and computer-readable representation of human knowledge and the derivation of new facts from it, but also with learning from experience (today usually through large amounts of annotated data).
This wealth of facets leads to a colorful bouquet of AI methods that can be profitably applied to a wide variety of real-world problems - in my case within agricultural engineering.
What was your personal path to becoming a professor at Hohenheim?
I began my academic career at the University of Applied Sciences in Augsburg, where I completed a bachelor's degree in business informatics. Because the theoretical content already gave me more at that time and I noticed, especially when studying for exams, that I was diving more and more into the theoretical background of the concepts being taught, I made the decision to complete my master's degree at a university.
So I did a master's degree in computer science and information management at the University of Augsburg. I didn't want to put the economic component completely aside. However, I have to admit that I limited myself to a minimum of credits in the economic subjects. Already towards the end of my bachelor studies, I came into contact with the concept of (software) agents -- an essential concept in AI -- for the first time.
In my first semester at university I had the choice between two modules that were offered at the same time. Funnily enough, the module I decided against at that time was the one that dealt more with machine learning methods, although it was called something completely different. Then, I found that a bit too mathematically formal even in the first lecture. Instead, I took a module called Organic Computing, which also gave a broader overview of nature-inspired methods of AI and their application in complex technical systems for use in the real world.
What can I say, I then started at the Department of Organic Computing as a research assistant, and over the next 5 years I became a PhD research assistant in computer science with a focus in rule-based machine learning.
During this time, I fully entered academia and learned a lot about and learned to love the academic work environment. It was clear to me that I wanted to stay in Academia and ultimately obtain a professorship. And now I'm here in beautiful Hohenheim and get to hold a tenure-track professorship in Artificial Intelligence in Agricultural Engineering - a dream come true.
That sounds nice. And what dream research topics are you working on at the moment?
We are currently working on a number of research topics in our research projects. These include very agricultural topics, such as AI-based optimization of power harrow intensity in the seedbed preparation process before sowing. But it also includes AI-based analysis of image recordings that map the visible and non-visible spectrum, for example, to detect plant diseases at early stages of infection. This is what we call "machine vision".
In another project, the focus is on AI method development for digitized agriculture. Here we deal with the automated creation and configuration of machine learning models (Automated Machine Learning), for example for crop yield forecasting. We also want to take into account the demand for data sovereignty. To this end, we are working on merging forecast models that are trained locally at different agricultural players - and without having to exchange the data in the first place.
Of course, intelligent and distributed field robotics also play an important role for us, even if initially more on a basic research level and in simulators.
Can students already participate in your research?
Yes, of course, we are already heavily involving students in our work. Last semester, for example, we had three students who very successfully completed the "Research Project" or "NawaRo Project" module at our department and were actively involved in our research projects.
Fachgebiet Künstliche Intelligenz in der Agrartechnik |
Seit dem 15.3.2020 leitet Jun.-Prof. Dr. Anthony Stein das neu eingerichtete Fachgebiet. Die Besetzung erfolgte erstmals im MWK-Programm Künstliche Intelligenz in Baden-Württemberg (KI-BW), mit dem das Land insgesamt 10 Juniorprofessuren zur KI finanziert. Die Professur mit Tenure Track ist auf 6 Jahre befristet und wird bei Bewährung in eine reguläre Professur umgewandelt. mehr |
We currently also offer topics for Humboldt Reloaded projects. Since the founding of the department, five students have successfully completed their theses. Four more master's theses are currently in progress. And of course, six HiWis are currently actively supporting us in our research projects.
What does good teaching mean to you?
For me, good teaching means, as a teacher, always being open to continuous learning. New, and in my particular case as a computer scientist at Hohenheim, perhaps sometimes "exotic" content should not simply be preached, but elicited together with the students and discussed again and again.
For this reason, I like to use interactive formats: Sometimes simply by means of small quizzes, sometimes completely seminar-style, and more recently flipped classroom style. In the latter format, students can, for example, deal with given guiding questions and scientific texts in preparation, and in the plenary session at the next session, these are discussed together and deepened.
However, it is not possible to teach theory completely, which is why I now like to use a mix of classic knowledge presentation (lecture) and the aforementioned flipped classroom model in my lectures. In this way, I try to convey the AI topics and teaching content, which often seem quite theoretical, intuitively at first with the help of smaller tangible examples, and to develop the more concrete applications of these concepts in agriculture together with the students.
Do you have any tips for successful study?
If a supposedly complex topic really interests you in your studies, then go for it and you will be able to learn it successfully. Many such topics can also be demystified somewhat. Particularly in AI, there are often simple but ingenious ideas behind it, which must first be grasped intuitively. After that, the rest often works itself out. Well, a little learning and practicing is always part of it, of course. And now I hope that many Hohenheim students who are basically interested in AI will read this tip ;-)
There's also life after university - what do you do when you're not working?
Every minute that I'm not working, I spend entirely with my little family and, of course, friends. Our two-year-old daughter keeps us pretty busy and her hobbies have naturally become my own. Unfortunately, I had to give up my Schafkopfstammtisch due to my move from Bavaria to Swabia.
That's hard to replace here, though... Thank you very much for the interview, Mr. Stein!
Interview: Elsner | Translation: International Office